Table of Contents (click to expand)
Moravec’s Paradox states that it is easy to train computers to do things that humans find hard, like mathematics and logic, but it is hard to train them to do things humans find easy, like walking and image recognition.
Computers assist in completing many high-level tasks that seem difficult for humans. We program them to do this by reverse-engineering the way we do it or making them learn on their own by feeding them trained data to determine better ways to achieve the objective (e.g., machine learning). Irrespective of the techniques, it is safe to say that computers have surpassed humans in doing certain tasks (e.g., playing chess, GO, analytics, etc.)
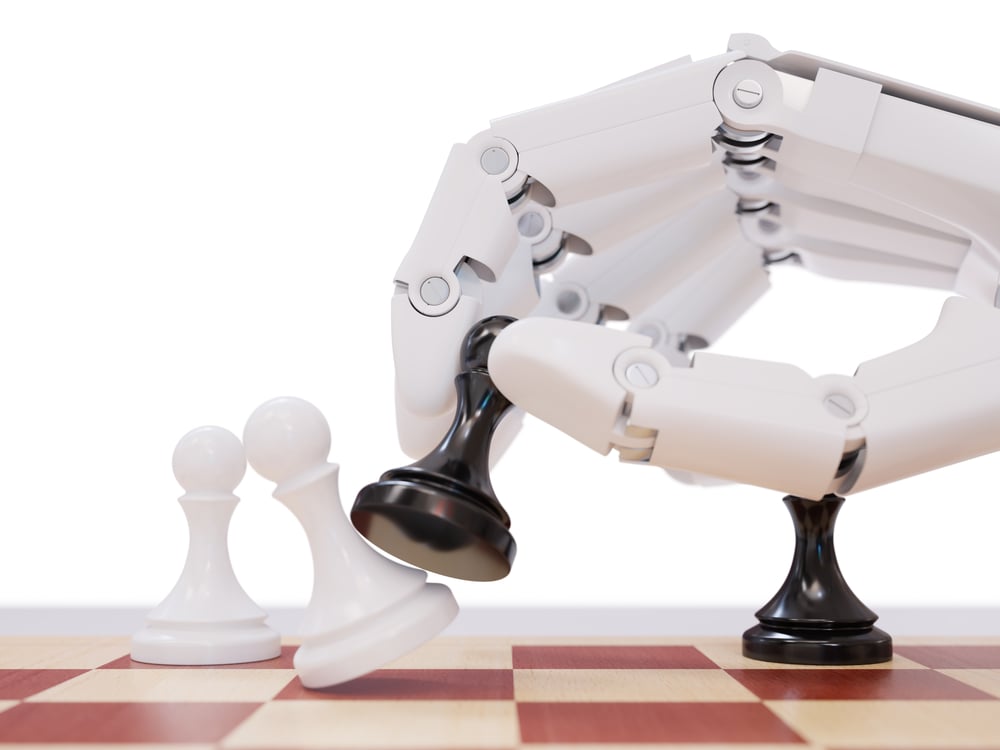
But in the 1980s, Hans Moravec and a group of AI researchers discovered something fascinating. They found that it is easy to train computers to do the things that seem hard to humans—playing chess, advanced statistics, algebra, etc. (all high-level adult stuff), but it was really hard to train them to do the things humans don’t even think twice while doing, such as identifying faces, perception, mobility, etc. (things even toddlers can do).
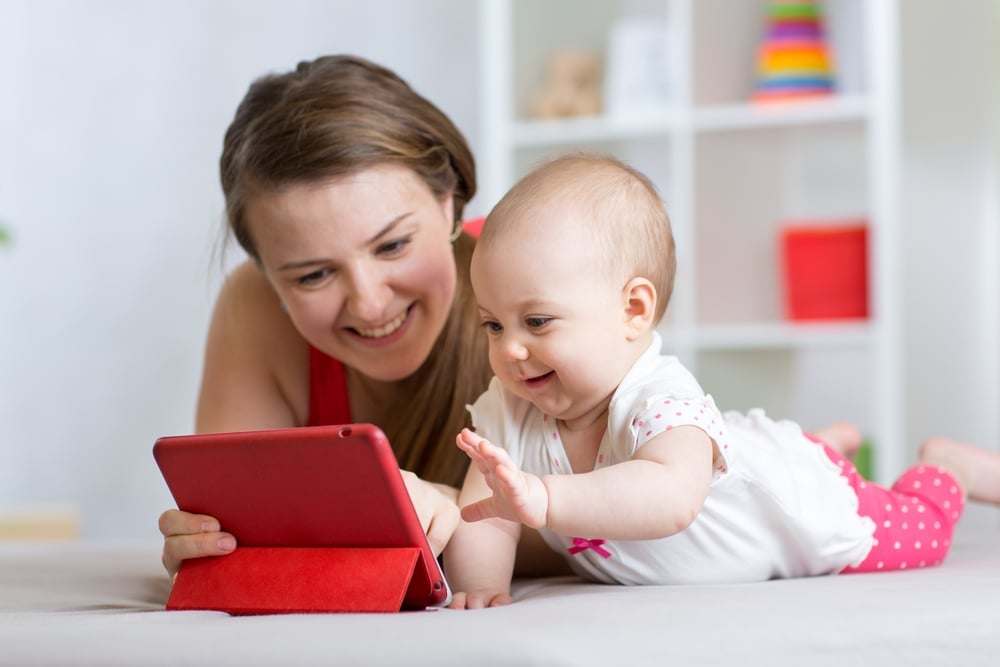
This came to be known as Moravec’s Paradox and it is one of the key concepts to understand the state of artificial intelligence.
Recommended Video for you:
The Paradox
Hans Moravec laid the basis of this paradox and considers one possible explanation to be rooted in evolution. In his book Mind Children, he reasons that we see this phenomenon—teaching computers hard things being easy and teaching them easy things being hard—is because machines are immune to the pressures of natural selection.
The hard things that we make computers solve are based on a deliberate process we call reasoning. This process is conscious, one of abstract thought and closely related to brain functions in humans that have evolved recently (less than 100,000 years ago) in the context of the long evolutionary process.
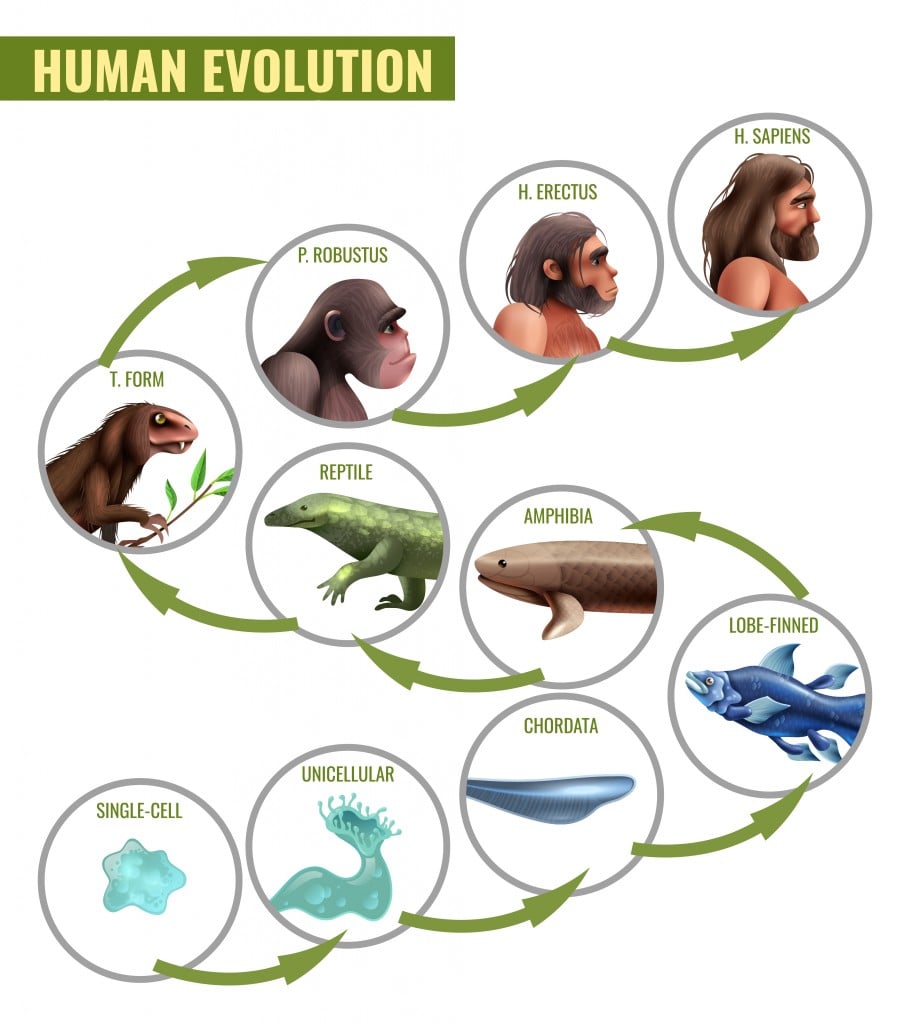
On the other hand, the easy things that we do, like walking, recognizing colors and faces, manipulative capabilities of the hand and other basic abilities have been ingrained over a long and arduous process of natural selection and evolution. These abilities that we possess are mostly unconscious and come to us without any thought; we seem to do them without any strain, making what looks difficult to be fairly easy. These unconscious processes are difficult to reverse-engineer and teach to the computers, thus increasing the complexity of the problem.
In short, the skills that humans have acquired recently in their history are easier to teach computers, but our skills get harder to teach as they go further back in the evolutionary history of humans and animals.
Also Read: Simulation Theory: Do We Live In The Matrix?
The Hard Problems That Are Easy
Computers are methodical and follow every step in the program to a tee; they can do this continuously without fail. This has enabled them to be very effective in the tasks that require repetitive execution of commands coded into them. They have become very effective in solving logic problems, algebra, geometry, and finding an ideal move in chess.
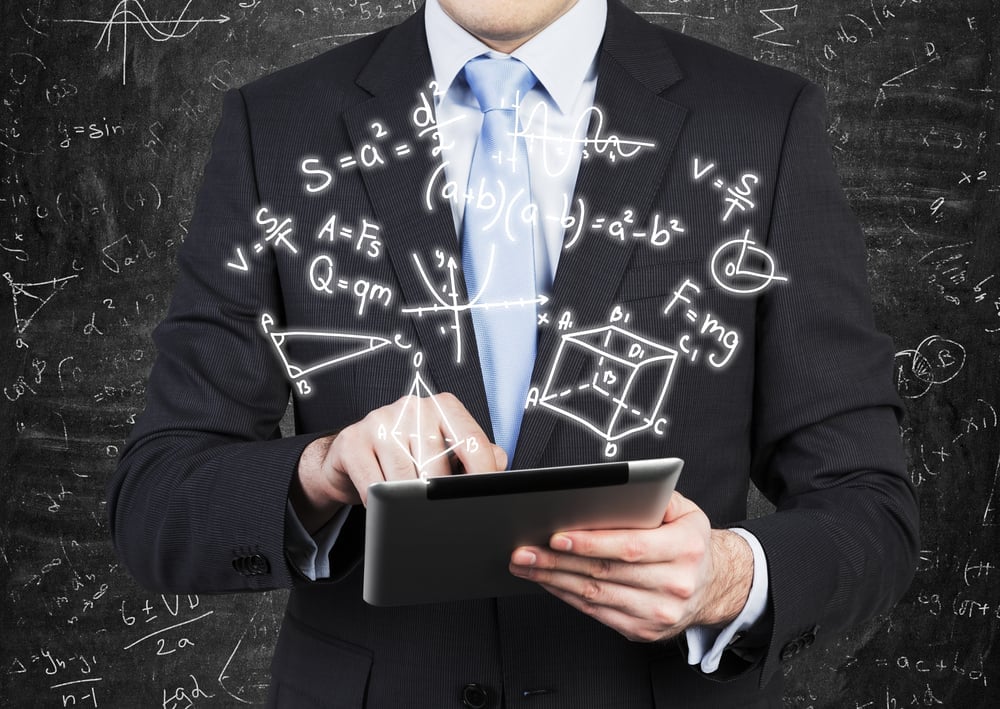
These problems are considered hard to solve for an average human. This has also been considered the definition of what being intelligent means—problems that are solved by highly trained scientists and those which even the most intelligent people find challenging.
These concepts, like mathematics and logic, are a recent development in the human skillset, making it easier for us to think about how we do them, reverse engineer the process, and then code it into a computer. Mastering these tasks has surely made computers better than humans in many ways, but it lags behind in more mundane things that humans accomplish every day. Making them able to do these things would create truly intelligent computers on par with humans.
Also Read: The Human Brain Vs. Supercomputers… Which One Wins?
The Easy Problems That Are Hard
Our sensory and perceptual functions have evolved over millions of years of trial and error and they can be traced back to single-celled organisms that started interacting with their environment. That is why tasks like walking, understanding visual clues, and interacting with the environment come so naturally and subconsciously to us.
These seemingly easy things for us are hard to teach to computers, but they are the bridge that computers must eventually cross for them to be on par with us. Many innovative ways are currently being developed, one that fundamentally involves making computers more adept at interacting with their physical environment.
With the ever-increasing improvements made in advanced sensors and cameras, computers are getting a more accurate picture of their immediate environment, helping them amass the right amount of data to make their decisions. With techniques involving modeling the neuronal pathways in the brain (deep learning), computers are getting continuously better in image and object recognition, sound-to-text translation, natural language processing, and other sensorial activities. Boston Dynamics has made great strides in highly mobile robots, which hint at what we can expect in the coming future.
Also Read: What Is Artificial Intelligence And How Is It Powering Our Lives?
Narrow And General Artificial Intelligence
These developments in making computer proficient for the hard problems that seem easy to us come from the field of Narrow AI. This specialty basically consists of training the computer in one very specific task, like recognizing cats in an image, mapping an environment for self-driving cars, natural language processing, and translation, etc.
Computers are being trained in creating art through these techniques as well, making them adept at every human skill set possible. Although we have yet to see a self-reflecting, creative, original thought-embodying artificial intelligence, projecting the current trends forward seems to indicate a point when computers will reach artificial general intelligence, putting them on par with a human.
If and when we do that, it will tell us whether we can truly get around this paradox and emulate millions of years of evolution in a fraction of the time.
References (click to expand)
- Moravec's Paradox. Brooklyn College
- H Hashmi —. ARTIFICAL INTELIGENCE - Washington. The University of Washington
- Artificial Intelligence (ANI & AGI) and its impact on society. Stanford University
- How will narrow Artificial Intelligence (narrow AI) change the .... Rutgers Business School – Newark and New Brunswick
- Moravec H. (1988). Mind Children: The Future of Robot and Human Intelligence. Harvard University Press