Table of Contents (click to expand)
Netflix uses data science to get you the right content all the time. They use a clustering and classification algorithm to classify all the current viewing content that people in your location have. They also use a recommender system to predict the future preference of a person given a fixed amount of limited data.
You come back home, turn on the TV and hop onto Netflix. The moment you log in, an array of exciting recommendations is presented to you. Chances are that you can jump right into picking content that you’d like to view; after all, there are so many ideal options for you right on the main page! However, have you ever stepped back and wondered how Netflix has such an array of movies, sitcoms and documentaries tailored so perfectly to your taste? Even if it’s not perfect for your palette, it’s often something that tickles your interest? Well to break down this answer, let’s first take a dive into understanding a bit more about data science.
Recommended Video for you:
Netflix And Data Science
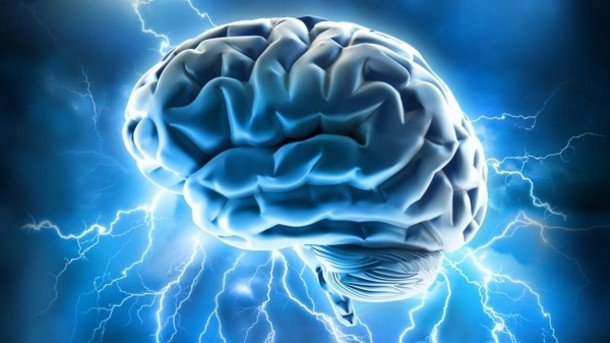
Data Science is a highly interdisciplinary field, consisting of statistics, probability and programming as the primary pillars. Data Science is primarily used to find patterns in large sets of data. It is usually employed to discern patterns that can give some underlying information about the data set being considered. Now, let’s try to understand how Netflix provides you with such great content from the get-go, i.e., when you have just joined their network. When you join the Netflix network, the company doesn’t have any information or data about your interests, likes or dislikes, so how exactly does the company provide that first assortment of entertainment options when it has no inkling of your tastes?
Well, the answer lies in what is known as a clustering and classification algorithm. What this does is quite ingenious. It classifies all the current viewing content that people in your location have. It is also guided by various parameters, such as your age versus the content commonly consumed by other people your age. This helps in clustering you into a specific preliminary array of entertainment that will later be fine-tuned by your usage patterns.
Also Read: How Are Movie Studios Using AI To Predict Movie Audiences’ Interests?
Recommender Systems
Once you start logging into Netflix regularly, you will realize that Netflix is usually spot on about what you’d like to see. This is done with the help of something known as a recommender system. A recommender system is a system capable of predicting the future preference of a person given a fixed amount of limited data. One primary reason Netflix uses a recommender system is the fact that a lot of content is generated for people that is entirely irrelevant to them based on their language or genres of interest.
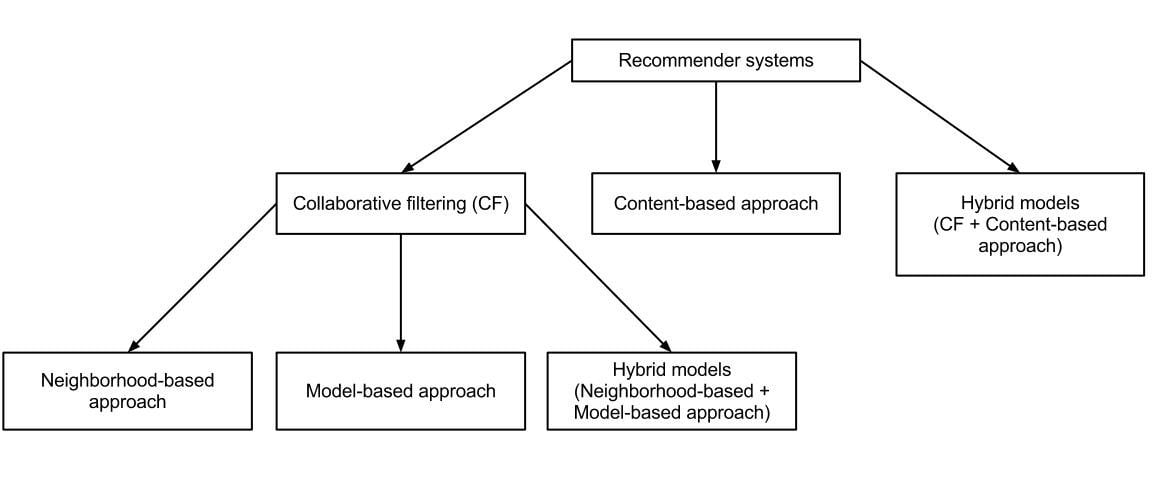
Content Based Recommendation
There are two ways in which a recommender system can be built. The first of these is known as a content-based recommendation. The idea of a content-based recommendation system is to filter the content based on a few parameters, such as likes, dislikes and viewing time. To understand this better, let’s take two movies, known as A and B. Now, let’s assume there are ten participants. If the participants like or dislike one movie over another, the recommender system would pick either movie A or movie B. However, that would be a perfect-case scenario where everyone leaves a rating. So what does one do when there is not a relevant amount of ratings to discern from for the recommender system? Well, that’s where the viewing time comes in. It can be safely assumed that if you like the content that you’re watching, you will watch it from start to finish. This can also be translated into data by the recommender system and used as an essential parameter in determining the likability of certain content.
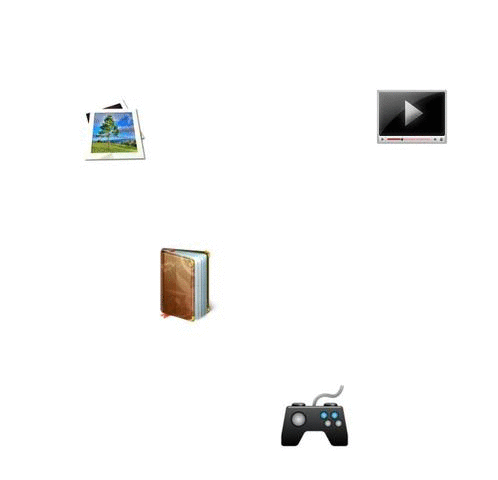
Collaborative Filtering
Another recommender system is known as collaborative filtering. Collaborative filtering is a method in which automatic predictions occur based on the collection of preferences and tastes within a pool of users. In a more general sense, collaborative filtering is the process of filtering for information or patterns using techniques involving collaboration among multiple agents, viewpoints, data sources, etc.
Applications of collaborative filtering typically require data sets of a substantial size. Collaborative filtering methods have been applied to many different kinds of data, including: sensing and monitoring data, such as in mineral exploration; environmental sensing over large areas or multiple sensors; financial data, such as financial service institutions that integrate many commercial sources; or in electronic commerce and web applications, where the focus is on user data, etc.
The underlying principle of collaborative filtering is that, if person A and person B like the same movie, then person A will likely have similar taste in movies as person B, rather than assuming that they might have similar tastes to a random person.
A slightly better way to utilize collaborative filtering is to employ a matrix method. Following this method, Netflix would create a group of movies and pair people together who have liked the maximum number of shared movies. Under this prediction method, people who fall under the same bracket are assumed to like the same movies in the future. In the end, we can say that the next time you sit down in front of your Netflix interface, you will understand that the movies proposed for your viewing pleasure have been closely considered and analyzed behind the scenes before they make your way onto your screen!
Also Read: Why Does Netflix Play The Trailer Of The Show/Movie When You Hover Over It?