Table of Contents (click to expand)
FaceApp uses Generative Adversarial Networks to train its program to create specific categories of realistic images. It then transfers the features to the photo uploaded by the user, giving it the desired category of the filter.
If you’ve been online lately, you have probably come across the viral challenge of aging your face 60 years into the future. Everyone from celebrities to your weird aunt seem to have hopped on this trend, which comes courtesy of FaceApp.
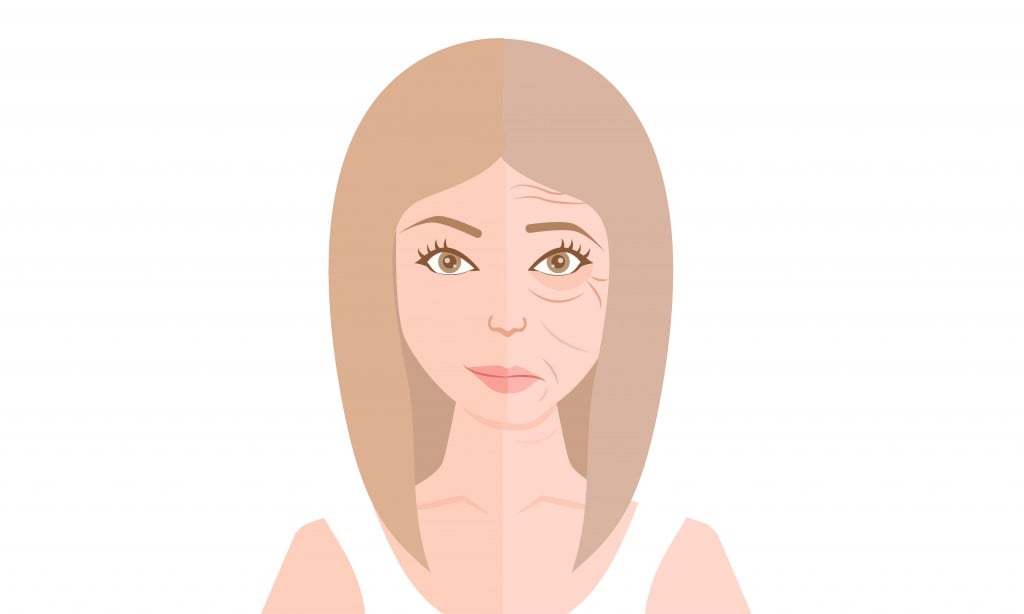
FaceApp is an image-manipulation application that enables users to post their selfies and retouch them in photorealistic alterations. One of the most famous uses of the app is to age your face into your 60’s and 70’s, essentially predicting how you’ll look later in life. It also enables gender-swapping, to see how you would look if your gender was reversed, in addition to enabling users to add features on your selfies, such as different beards or hairstyles.
The features have garnered a lot of mainstream attention and rightfully so, as the results are very realistic and occasionally unnerving. How do they manage to achieve such highly precise, on-the-spot image manipulation? Let’s find out!
Recommended Video for you:
Background
FaceApp was created by Wireless Lab and initially released in January of 2017. The app mainly focuses on selfies or photos with faces; transforming them to make the image smile, look older, look younger, envision additional features (hairstyles, beard) or change the gender.
FaceApp went viral in 2017, as people began posting challenges to transform their selfies with the app’s features. The image results of the app are very convincing—and entertaining—which helped the app spread like wildfire on Facebook and Instagram. The app has now been downloaded more than 100 million times, making it the top-ranked app on the iOS App Store in 121 countries.
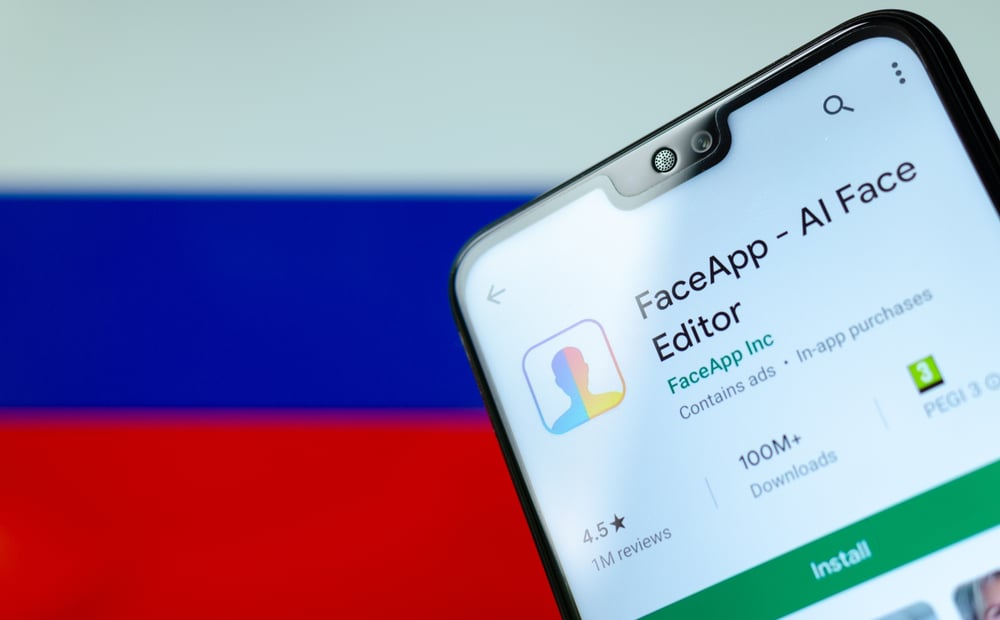
The app uses neural networks to quickly manipulate the images. The results are truly something to behold, as it is very difficult to discern an altered image from reality. The app gives some glimpse into the prowess of artificial intelligence in image and video manipulation, employing tested methods to garner app growth.
How Does It Work?
FaceApp uses machine learning to make its program manipulate user-fed images. These photos come from your library or are snapped within the app. The app provides various manipulation features in both its free and paid versions. Once transformed and saved, you can then post the images to the platform of your choosing—Facebook, Instagram, WhatsApp, etc.
FaceApp uses a very specific type of machine learning technique called a neural network. These networks are modeled on the neuronal processes of the human brain and can be trained to recognize and manipulate specific tasks. The training data goes through multiple layers—much like the brain—from which it extracts specific features that make up the particular set of data.
To enable the the program to recognize faces and add modifications to get the desired effect, FaceApp uses a subset of neural networks called Generative Adversarial Networks (GAN).
Generative Adversarial Networks (GAN)
GAN’s operate two neural networks pitted against each other to create a realistic image. One of the networks is called a generator and its job is to take noise vectors (a list of random numbers) and generate an image. These random numbers ensure variation in the generated image so that it produces a different image every time.
The second network is called a discriminator and its job is to critique the images created by the generator. The discriminator critiques the images on the basis of the real-world data it is fed. It will continually reject the images and provide feedback on the parts that are flawed. Given enough time and computation power, the generator will eventually pass all the criteria of the discriminator and create a realistic image.
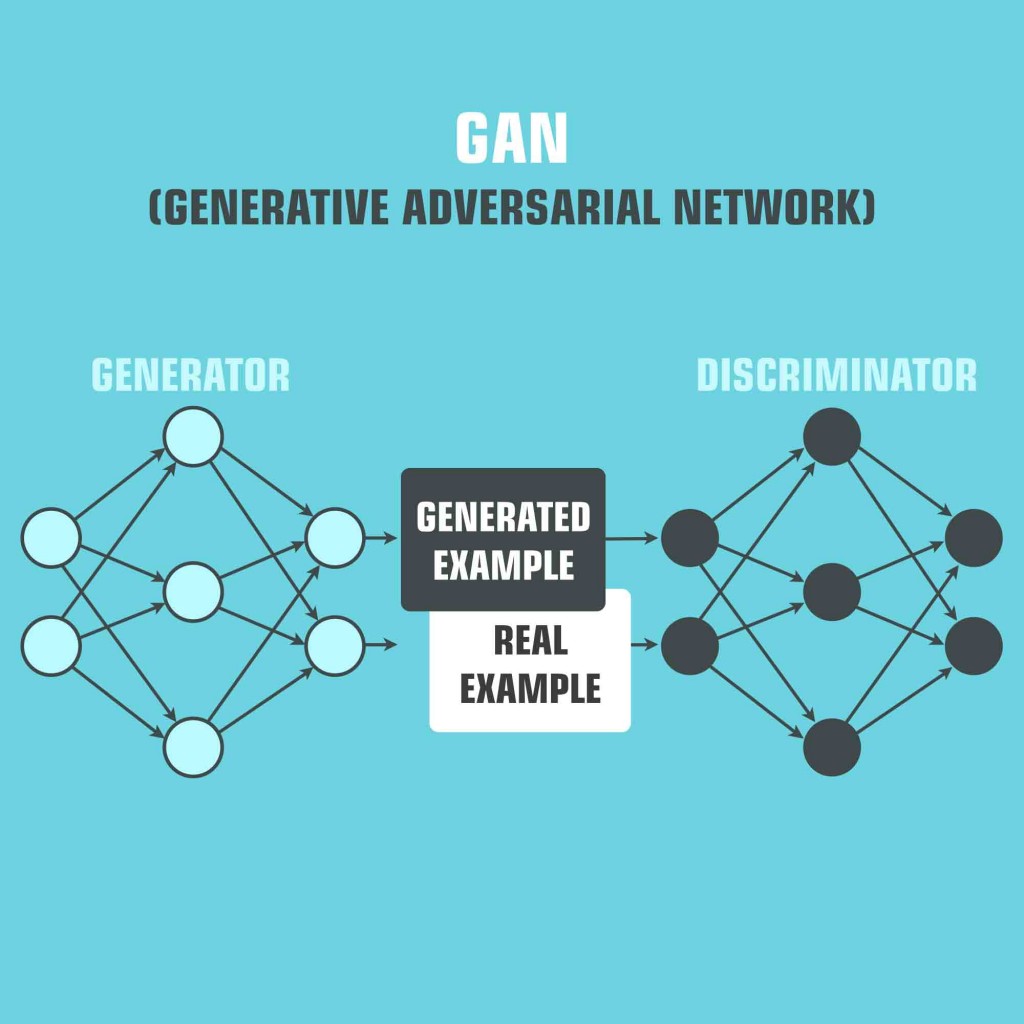
To create images with specific features, the GAN goes a step further and a condition is added. For example, to get the network to generate photos of elderly faces, it is fed training data labeled by age, which helps the GAN know the characteristics.
The generator then generates photos of various ages and the discriminator critiques them on the basis of the training data. The condition is changed for another feature (creating young faces) and the procedure is repeated. Thus, when you take a selfie and select the option to make your face look older, the app transfers the trained feature to the image from its huge training library, while maintaining the primary aspects of the person, but giving them ‘oldness’.
Controversy
Although the app showcases great image manipulation capabilities, it has been frequently surrounded by controversy. During its initial release, the app was scrutinized for having a filter that made photos of people ‘hot’ by making their skin tone fairer. The app also came into an unwanted limelight for their filters that changed people’s faces into ‘Black’, ‘Indian’ and ‘Asian’ and critics slammed the app for being racist.
As the app gained more attention and went insanely viral on various platforms, other controversies began to loom. Many users had concerns over the privacy of their submitted data and the unfettered access that FaceApp has to the images in the phone on which it is installed. The app has also been accused of selling the thoroughly labeled data garnered from their millions of users to third-party companies eager to access such data.
However, these controversies haven’t seemed to stop the virality of the app, as it keeps gaining more followers every day. It seems to have touched on a curious nerve of users and is likely to keep updating its features to stay relevant and continue dominating app marketplaces around the world.
References (click to expand)
- Up‐Sampling Artifact & GAN Pipeline Emulator. Columbia University
- Deepfake Video Detection Using Recurrent Neural Networks - engineering.purdue.edu
- We can't get enough of FaceApp. But should we be giving .... Northeastern University
- Viral App FaceApp Now Owns Access To More Than 150 Million People's Faces And Name | UCLA IT Services - www.it.ucla.edu